AI Security - University of KZN MSc Lecture Series (part 1 of 4)
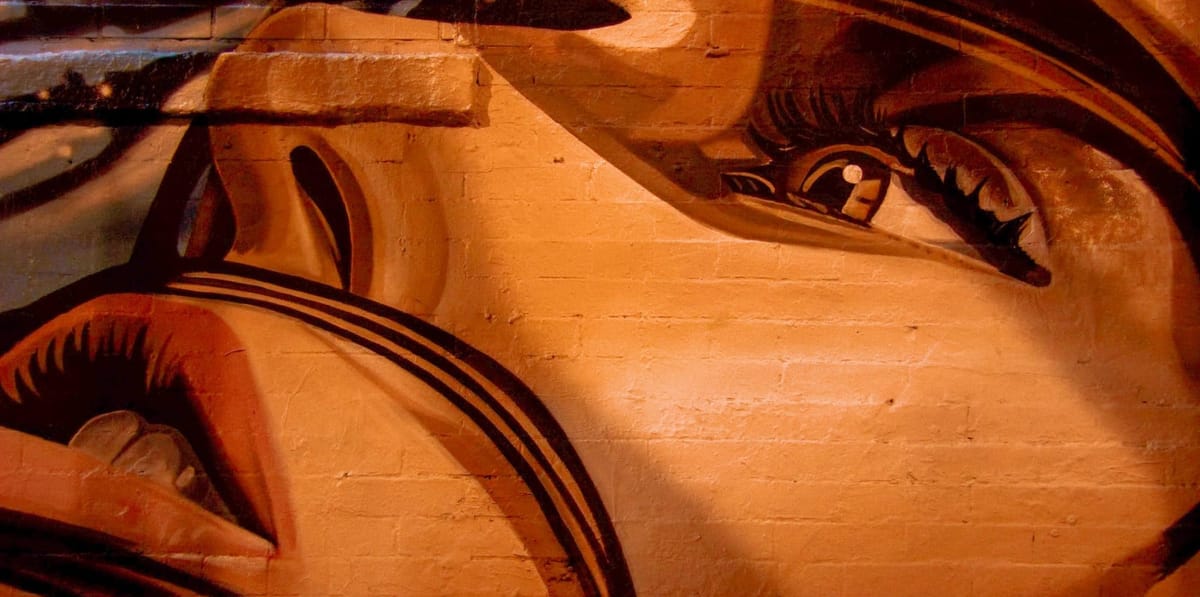
Last month I was invited to give a 2-part lecture on AI Security at UKZN. This was a big honour for me - since I'm a fan of their AI and Quantum researchers (e.g., Amira).
Thanks to Prof Manoj Maharaj, for inviting various industry guest lecturers to UKZN. Upcoming speakers include numerous global thought leaders (e.g., Prof Petruccione).
The 3-hour lecture was divided into 4 components - which I will cover in 4 blog posts:
- Defensive AI;
- Offensive AI;
- AI Security; &
- Responsible AI.
I recommend reading the article with the original slides - which you can get here.
AI Security
Let's start with AI Security, since I ran out of time and couldn't complete the section.
This article covers the following:
- Scope of assessment;
- Types of assessment;
- Review components;
- Your requirement; &
- Review methodology.
Scope of AI security assessment
At Snode Technologies we often need to perform security assessments on AI designs, implementations and existing AI operations (e.g., AI red team testing). This includes:
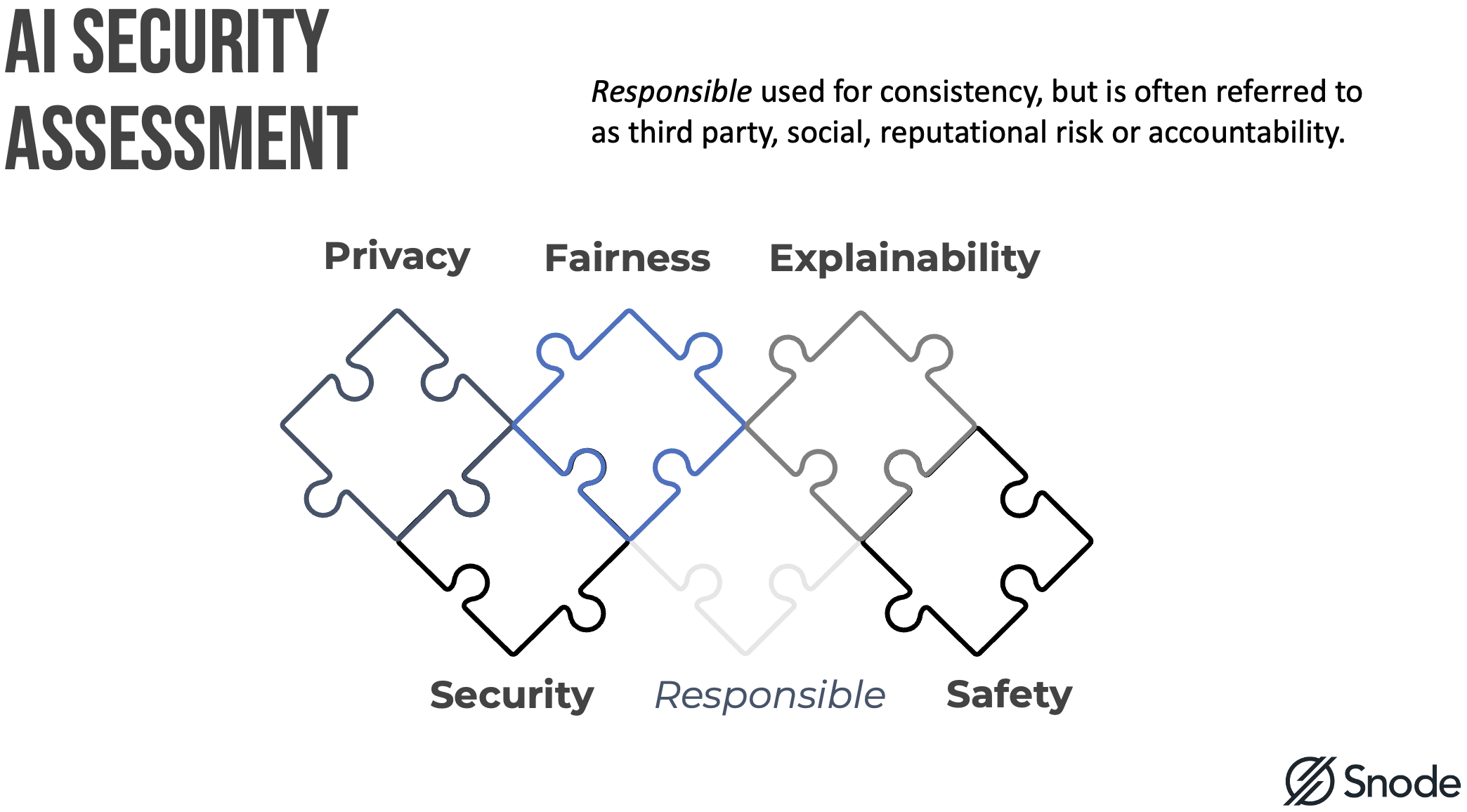
You (the student) should also look at other framework's scope for secure AI adoption.
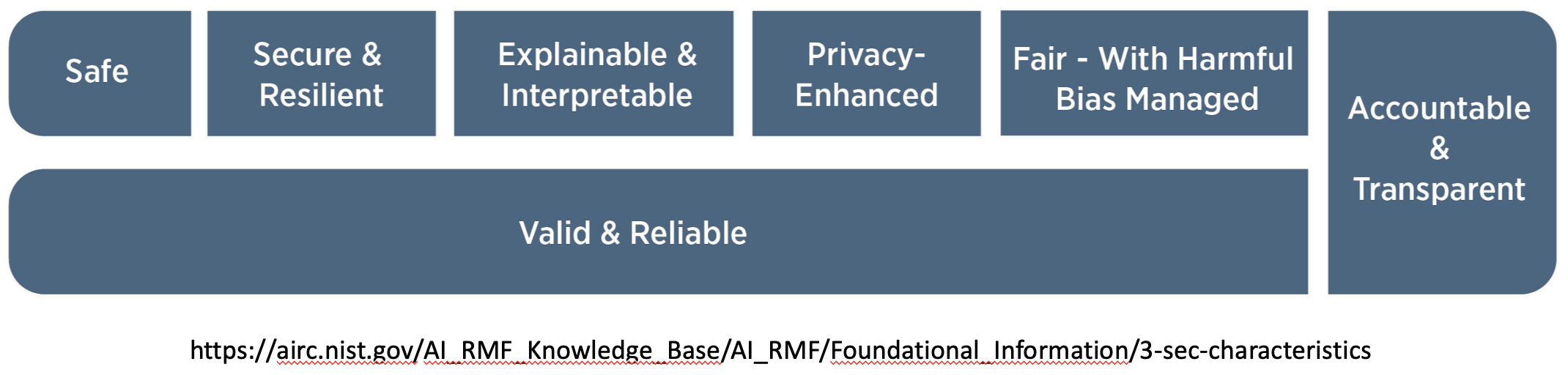
Types of AI Security assessments
There are three (3) types of assessments we offer Snode clients, each with unique strengths and applicable to where you are in your AI development lifecycle, namely:
- AI Design Review;
- AI Red Teaming; &
- AI Risk Assessment.
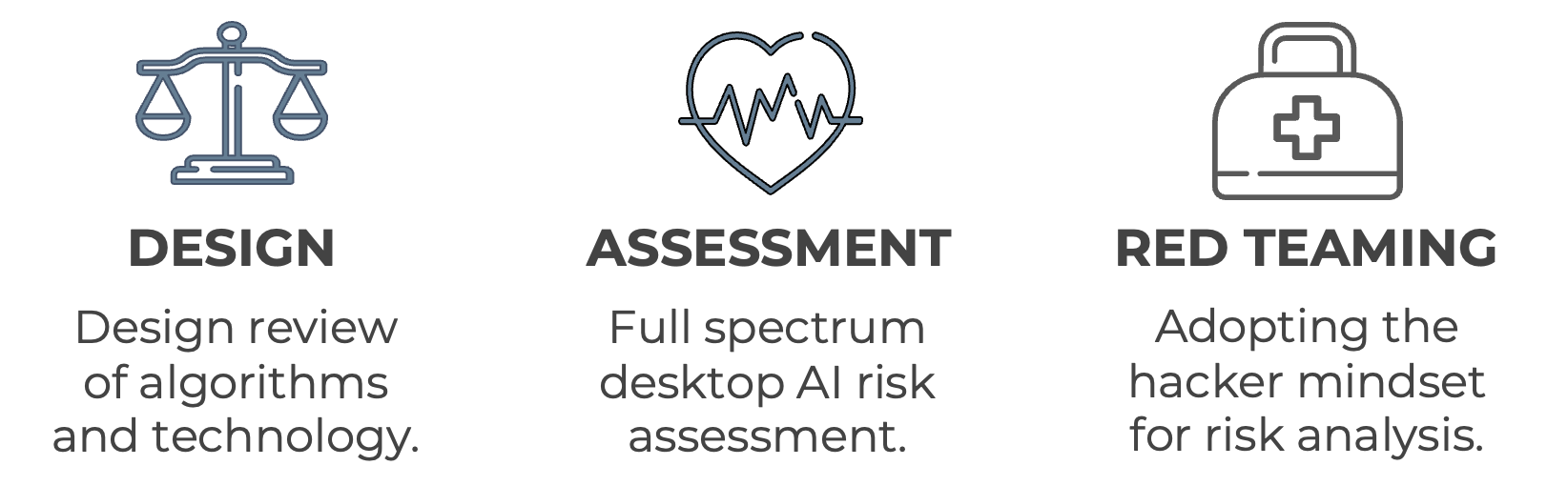
I will do a future series of articles, covering the methodologies for each, in more detail.
AI Security - components for review
Here are some high-level control areas we will look to cover during these assessments. This highlights the full spectrum of issues that are covered for a comprehensive review.
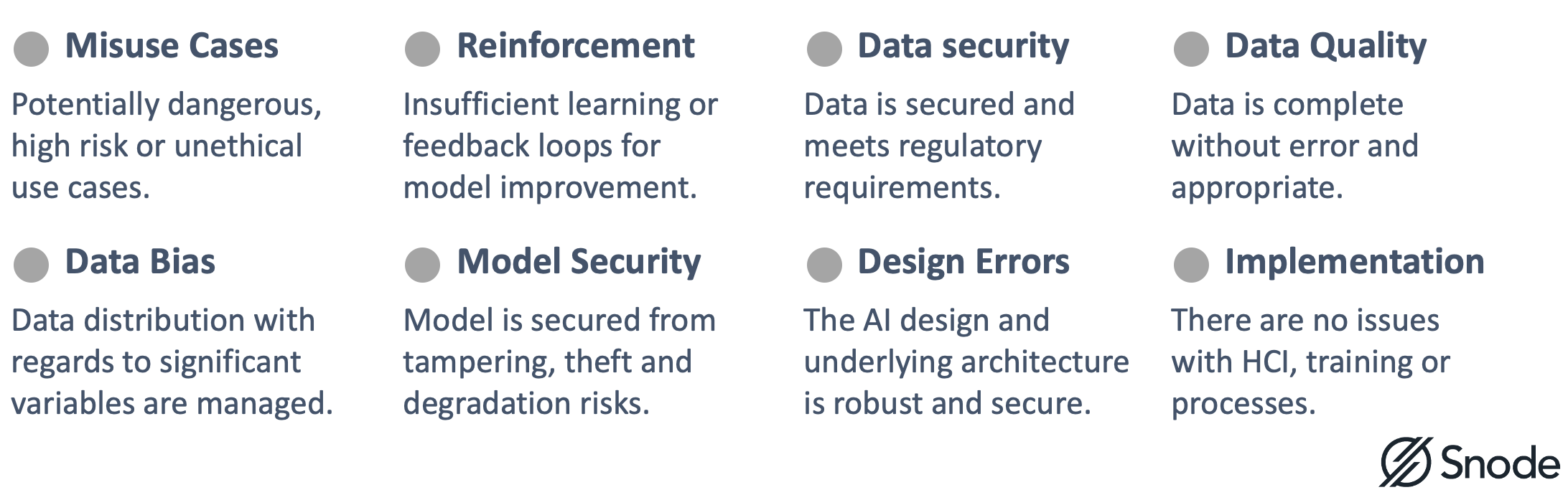
Understanding the AI requirements
As with any assessment there are three forces driving your security requirements.
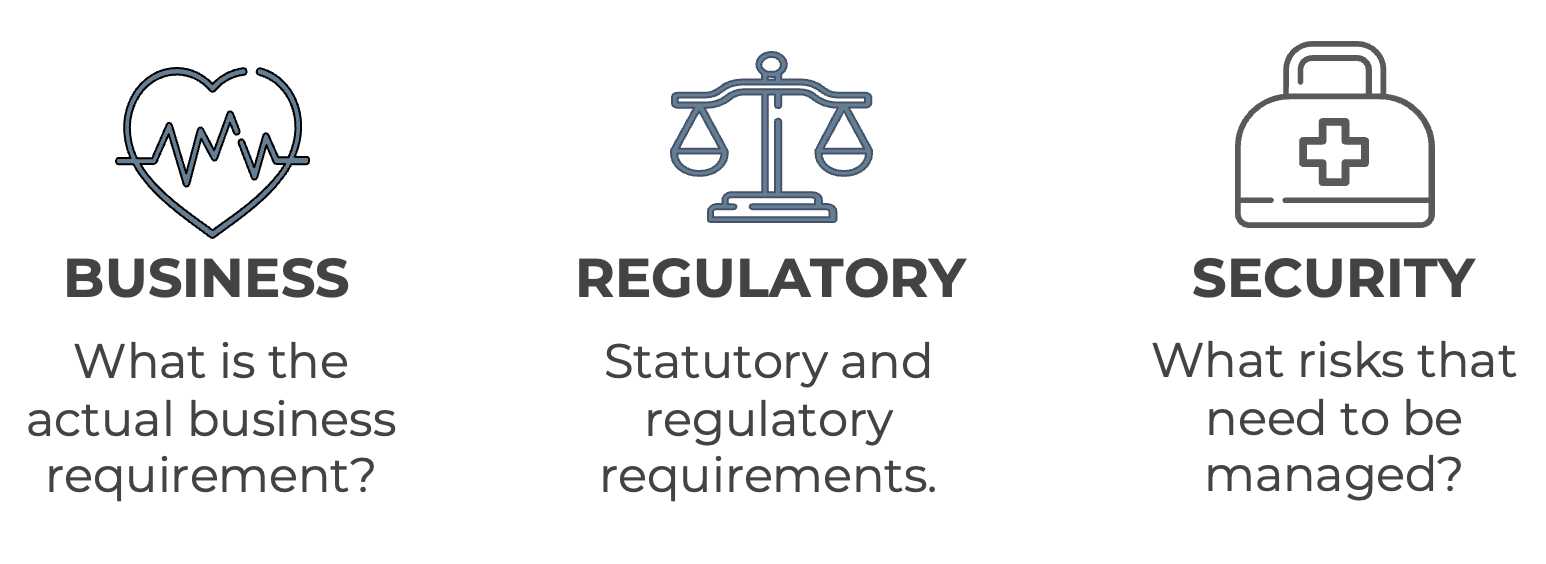
So why assess alignment to the business requirement? Well, one of the most common overlooked risks with clients - is model drift. Model drift occurs when a model doesn't align or moves out of alignment over time (degradation) with the business objective(s).
Regulatory and statutory requirements, such as the EU AI ACT, need to be addressed.
Security requirements need to address the risks associated with the environment, area and context of the AI application. Here are a few high-level examples:
- Is there a risk to human life? Such as an autonomous vehicle use case.
- Is there potential for abuse? Such as misinformation or disinformation.
- Is there a privacy concern? Such as facial or biometric data disclosures.
High-level AI review methodology
At a high-level the review methodology consists of the following activities:
- Business - strategic objective and application alignment.
- Culture - AI change management, AI training and talent.
- Compliance - AI/ IS regulatory and statutory requirement.
- Model specific risk - tampering, drift, poisoning, theft, etc.
- Infrastructure - underlying or supporting technology risk.
- Data risk - with collection, transmission, storage, bias, etc.
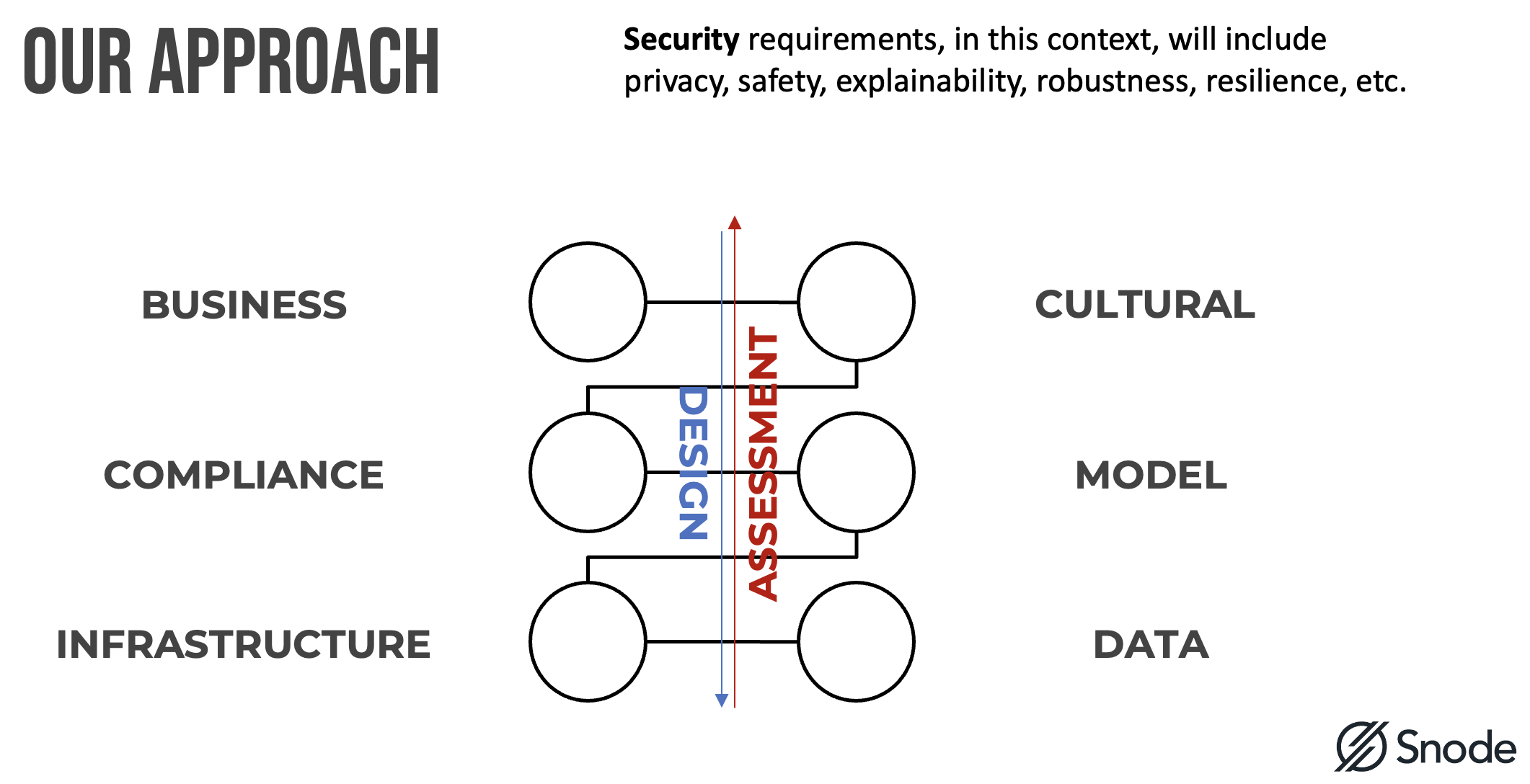
If we perform a AI design review, we tend to move downwards, starting with the business requirement. However, for Red Teaming, we move upwards - starting with operational effectiveness and then perform a root-cause analysis with earlier stages.
I recommend to clients that they perform all three assessments at the appropriate point in the AI development lifecycle (secure design directly after the design phase).
Disclaimer - if I got something wrong,...
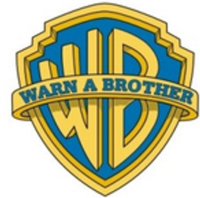
AI Assessment References

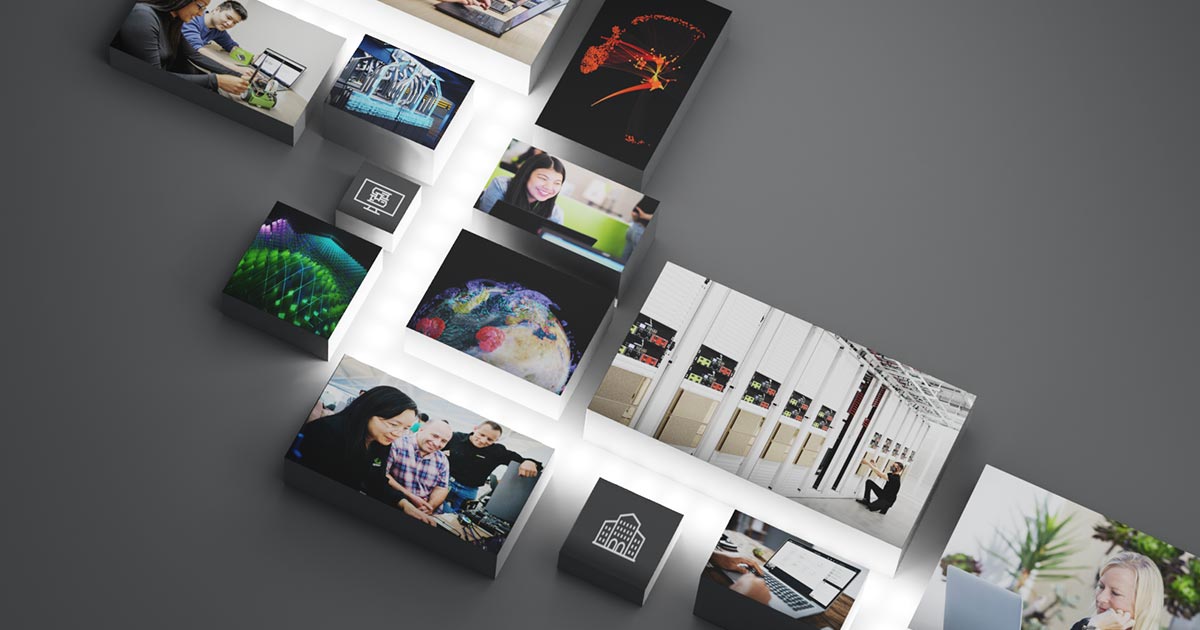
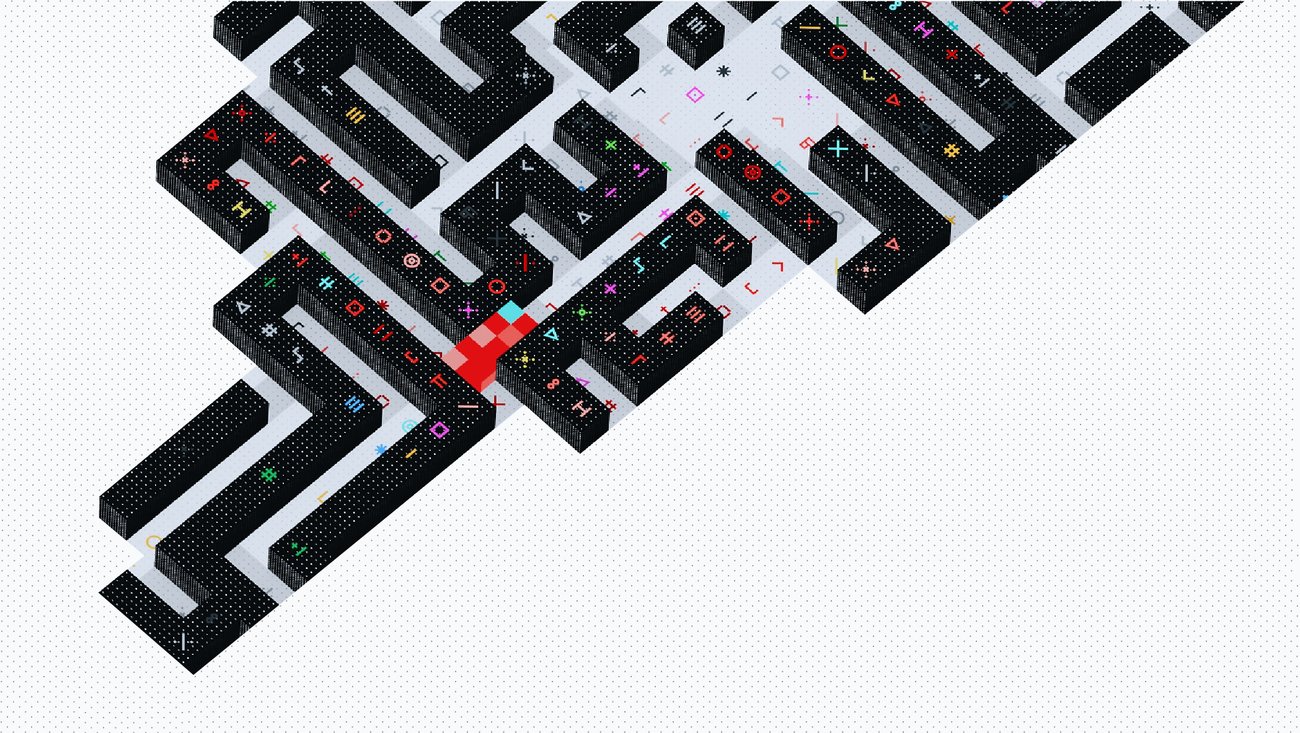